Lab researchers win top award for machine learning-based approach to ICF experiments
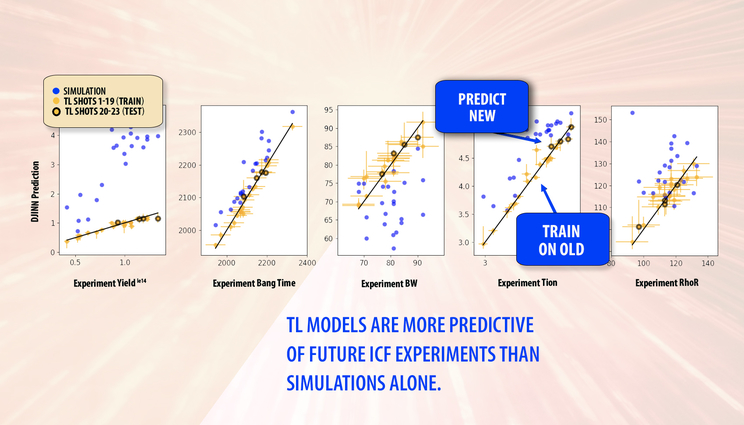
The figure plots actual values (x-axis) versus predicted values (y-axis) for five measured quantities of interest in Omega ICF experiments — the neutron yield, bang time, burnwidth, ion temperature and areal density.
The IEEE Nuclear and Plasma Sciences Society (NPSS) announced a Lawrence Livermore National Laboratory (LLNL) team as the winner of its 2022 Transactions on Plasma Science (TPS) Best Paper Award for their work applying machine learning to inertial confinement fusion (ICF) experiments.
In the paper, lead author Kelli Humbird and co-authors propose a novel technique for calibrating ICF experiments by combining machine learning with experimental data via “transfer learning” (TL), a method in which models are trained on a task and then partially retrained to solve a separate, but related task with limited data.
The team, which includes Luc Peterson and Brian Spears of LLNL and Ryan McClarren from the University of Notre Dame, introduced the concept of hierarchical TL, where neural networks trained on low-fidelity models are calibrated to high-fidelity models and then applied to experimental data. The researchers applied the technique to a database of ICF simulations and experiments carried out at the University of Rochester’s Omega Laser Facility, finding the combination of deep neural networks with experiments resulted in better and more predictive models of ICF experiments than simulations alone.
Typically, ICF experiments are designed using computer simulations that approximate physics modeling and must be calibrated to predict experimental observations, usually through a statistics-based Bayesian method.
“In this paper, we were really able to pioneer the use of transfer learning as a way to calibrate scientific simulations,” Humbird said. “Transfer learning is a fundamentally different approach to calibrating a simulation-informed model to experimental data, and it works well in some cases where the Bayesian approach struggles.”
Along with the award, Humbird and team received a plaque, certificates and a cash prize of $1,500 divided equally among team members. The work is part of a broader Department of Energy effort to discover, design, manufacture and deploy artificial intelligence and machine learning into every stage of a project. With a more reliable, AI-driven simulator, researchers could predict future experiments at the National Ignition Facility and find new optimal implosion designs, Humbird explained.
“Simulators are good maps, but transfer learning helps you take that good map and pinpoint real, experimentally observed features by taking into account all the experimental data we’ve gathered in our ICF implosions and folding them into the model,” Humbird said. “The transfer learning model also gets smarter over time, constantly updating its idea of what the ‘NIF map' looks like as we fire more and more shots at the facility. So, when it comes to design optimization, using this data-informed model to search for designs holds promise, since we have confidence that the map we’re using to chart our path to high performance is accurate.”
In addition to fusion research, transfer learning could have impacts beyond ICF, including any experiment that uses approximate simulations to model complex systems and requires a predictive model to be generated with only a small amount of experimental data.
The annual award is the fourth of its kind handed out by the IEEE TPS journal, which covers the theory and application of plasma science and engineering and disseminates technical information for the IEEE NPSS’ Plasma Science and Applications Committee, Pulsed Power Science and Technology Committee, and Fusion Technology Committee.
The journal determined the award-winner based on criteria including: quantifiable usefulness to the community, the number of downloads, citations to the paper, quality, clarity of presentation, originality, significance and contributions to the field.
The award will be presented at the Conference on Plasma Science in Santa Fe, New Mexico, in May 2023.
Contact

[email protected]
(925) 422-5539
Related Links
IEEE Nuclear and Plasma Sciences Society"Transfer Learning to Model Inertial Confinement Fusion Experiments"
Tags
HPC, Simulation, and Data ScienceComputing
Engineering
Lasers and Optical S&T
Lasers
National Ignition Facility and Photon Science
Featured Articles
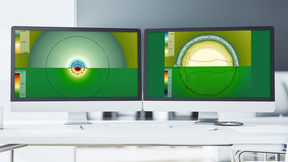
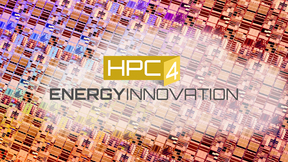
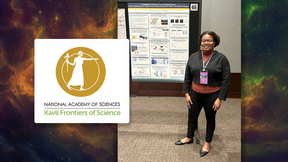