Lab scientists and engineers garner three awards for top inventions in R&D 100 competition
Lawrence Livermore National Laboratory (LLNL) scientists and engineers have netted three awards among the top 100 inventions worldwide.
The trade journal R&D World Magazine recently announced the winners of the awards, often called the “Oscars of innovation,” recognizing new commercial products, technologies and materials that are available for sale or license for their technological significance.
With this year’s results, the Laboratory has now collected a total of 179 R&D 100 awards since 1978. The awards will be showcased at the 61st R&D 100 black-tie awards gala on Nov. 16 in San Diego.
This year’s LLNL R&D 100 awards include a software suite that helps apply deep-learning techniques to major science and data challenges in cancer research; software that helps better understand the power, energy and performance of supercomputers; and a number format that permits fast, accurate data compression for modern supercomputer applications.
All three of LLNL’s R&D 100 award winners received internal “seed money” from the Laboratory Directed Research and Development program. This funding enables the undertaking of high-risk, potentially high-payoff projects at the forefront of science and technology.
“The R&D 100 awards highlight the most game-changing technologies each year,” Lab Director Kim Budil said. “Researchers at LLNL strive to address the most significant challenges facing the world today through innovative science and technology and these awards are an important recognition of the impact of this work.”
Software aids scientific discovery
Supercomputers can enable remarkable research and scientific discoveries, but they require sophisticated coordination of application codes, diverse hardware components and system software.
Efficient systems can process more user requests, improve the pace of scientific discovery and help better understand power and energy costs, allowing for better utilization in supercomputing practices.
A team of LLNL computer scientists has developed Variorum, a vendor-neutral software library for exposing and monitoring the power, energy and performance of low-level hardware dials across diverse architectures in a user-friendly manner for supercomputers.
It is part of the Department of Energy’s (DOE) Exascale Computing Project (ECP), specifically the Argo Project in which Variorum is a key component for node-level power management in the high-performance computing (HPC) PowerStack Initiative.
Variorum focuses on ease of use and reduced integration burden in scientific applications and workflows. It has enabled support for all three upcoming U.S. exascale supercomputers — El Capitan at LLNL, Aurora at Argonne National Laboratory and Frontier at Oak Ridge National Laboratory — and many other HPC systems.
The Variorum team is led by Lab computer scientist Tapasya Patki and includes computer scientists Aniruddha Marathe, Barry Rountree, Eric Green, Kathleen Shoga and Stephanie Brink.
Accelerating data movement for supercomputers
A team of LLNL researchers has developed ZFP, an open-source software that compresses numerical data exceptionally fast and allows reductions in data movement, something that is critical to today’s high-performance computing (HPC) applications. HPC performance is largely limited by data movement rather than raw compute power.
ZFP allows computer users to store numerical data using less space, so that they can cram larger data sets into memory or reduce how much disk space they need. ZFP also allows users to transfer data, such as between memory and disk, between different compute nodes on a supercomputer and across the internet, more quickly by having the sender compress it, transmit the data in reduced form and have the receiver decompress it in order to reconstruct the original data.
ZFP effectively expands available central processing unit and graphics processing unit memory by as much as 10-fold; reduces offline storage by 10-fold to 100-fold; and — by reducing data volumes — speeds up data movement.
Contrary to competing compressed formats designed for file storage, a unique feature of ZFP is that it supports high-speed random access to array elements — both for read and write operations — suitable also for in-memory storage.
ZFP was developed by a team of LLNL researchers led by computer scientist Peter Lindstrom and includes computer scientists Danielle Asher and Mark C. Miller. In addition, three former Lab employees — Stephen Herbein, Matthew Larsen and Markus Salasoo — who have since left LLNL were part of the team.
CANDLE aids cancer patients
The ECP-CANDLE Project (Exascale Computing Project — CANcer Distributed Learning Environment) brought together the combined resources of the DOE and the National Cancer Institute to accelerate cancer-specific research by applying machine learning and deep learning techniques to large-scale cancer datasets in a distributed computing environment.
The resultant software suite, called CANDLE, provides cancer researchers with key functions and capabilities so they can study molecular interactions using dynamic simulations and predict responses to treatment as well as patient trajectories and outcomes.
As part of the five-lab consortium, this work led to a specific focus for the LLNL team within CANDLE on the development of scalable distributed deep learning tools, algorithms and methods within the open-source Livermore Big Artificial Neural Network toolkit (LBANN) and targeting the development of neural network models representing the state transition of the RAS-RAF protein complex as it binds to a lipid membrane.
Several of the key innovations developed by the LLNL research team were the creation of a scalable deep learning tournament algorithm, scalable distributed in-memory data storage optimized for deep neural network training, improved methods for tensor and model parallelism, as well as the capability for dynamic tuning of compute kernels that are optimized for next generation HPC hardware architectures.
Additionally, the CANDLE project and LLNL team worked to enable external deep learning toolkits, such as PyTorch, DeepSpeed, and Megatron-LM, to run on the first generation of exascale computers as well as help optimize their performance on these systems.
The CANDLE collaborators are: Argonne, Oak Ridge, Lawrence Livermore and Los Alamos national laboratories as well as Fredrick National Laboratory for Cancer Research.
The LLNL part of the ECP-CANDLE team is led by Lab computer scientist Brian Van Essen and chief computational scientist Fred Streitz and includes current team members Tom Benson, Adam Moody, Tal Ben-Nun, Nikoli Dryden, and Pier Fiedorowicz as well as former Lab employees David Hysom, Sam Ade Jacobs, Naoya Maruyama and Tim Moon.
Contact
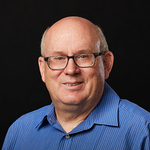
[email protected]
(925) 423-3107
Related Links
R&D World MagazineLivermore researchers collect three awards among the top 100 industrial inventions
Tags
HPC, Simulation, and Data ScienceComputing
Economic Impact
Industry Collaborations
ASC
Science
Technology Transfer
Featured Articles

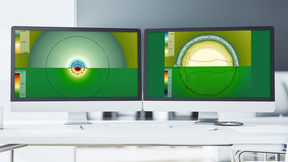
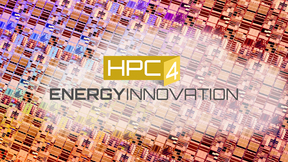