Come on in, the water is superionic
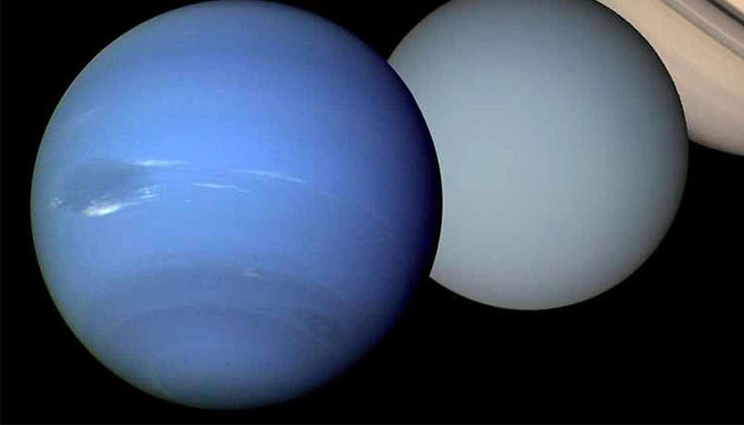
LLNL scientists have developed a new approach using machine learning to study with unprecedented resolution the phase behaviors of superionic water found in ice giants Uranus and Neptune.
The interiors of Uranus and Neptune each contain about 50,000 times the amount of water in Earth’s oceans, and a form of water known as superionic water is believed to be stable at depths greater than approximately one-third of the radius of these ice giants.
Superionic water is a phase of H2O where hydrogen atoms become liquid-like while oxygen atoms remain solid-like on a crystalline lattice. Although superionic water was proposed over three decades ago, its optical properties and oxygen lattices were only accurately measured recently in experiments by LLNL’s Marius Millot and Federica Coppari, and many properties of this hot “black ice” are still uncharted.
Lawrence Livermore National Laboratory (LLNL) scientists have developed a new approach using machine learning to study the phase behaviors of superionic water with unprecedented resolution.
Buried deep within the core of planets, much of the water in the universe may be superionic. Understanding its thermodynamic and transport properties is crucial for planetary science but difficult to probe experimentally or theoretically.
Under the pressures and temperatures found in ice-giant planets, most of this water was predicted by First-Principles Molecular Dynamics (FPMD) simulations to be in a superionic phase. However, such quantum-mechanical simulations have traditionally been limited to short simulation times (10s of picoseconds) and small system size (100s of atoms), leading to significant uncertainty in the location of phase boundaries such as the melting line.
In experiments on superionic water, sample preparation is extremely challenging: hydrogen positions cannot be determined and temperature measurements in dynamical compression experiments are not straightforward. Often the experiments benefit from the guidance provided by quantum molecular dynamic simulations both during the design stage and for the interpretation of the results.
In the most recent research, the team made a leap forward in its ability to treat large system sizes and long-time scales by making use of machine learning techniques to learn the atomic interactions from quantum mechanical calculations. They then used that machine-learned potential to drive the molecular dynamics and enable the use of advanced free energy sampling methods to accurately determine the phase boundaries.
“We use machine learning and free energy methods to overcome the limitations of quantum mechanical simulations, and characterize hydrogen diffusion, superionic transitions and phase behaviors of water at extreme conditions,” said LLNL physicist Sebastien Hamel, a co-author of a paper appearing in Nature Physics.
The team found that phase boundaries, which are consistent with the existing experimental observations, help resolve the fractions of insulating ice, different superionic phases and liquid water inside of ice giants.
The construction of effective interaction potentials that retain the accuracy of quantum mechanical calculations is a difficult task. The framework that was developed here is general and can be used to discover and/or characterize other complex materials such as battery electrolytes, plastics and nanocrystalline diamond used in Inertial Confinement Fusion (ICF) capsules, as well as new phases of ammonia, salts, hydrocarbons, silicates and related mixtures that are relevant for planetary science.
“Our quantitative understanding of superionic water sheds light into the interior structure, evolution and magnetic fields of planets such as Uranus and Neptune and also of the increasing number of icy exoplanets,” Hamel said.
Researchers from the University of Cambridge, the University of Lyon and Tohoku University also contributed to the paper. The LLNL portion of the research is funded by the Laboratory Directed Research and Development project "Unraveling the Physics and Chemistry of low-Z Mixtures at Extreme Pressures and Temperatures" and the Institutional Computing Grand Challenge program.
Contact
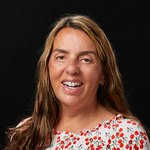
[email protected]
(925) 422-9799
Related Links
Experimental evidence for superionic water ice using shock compressionNanosecond X-ray diffraction of shock-compressed superionic water ice
Nature Physics
Tags
PhysicsPhysical and Life Sciences
Science
Featured Articles
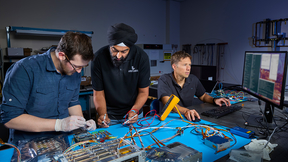

