Kamath offers practical resource on data mining
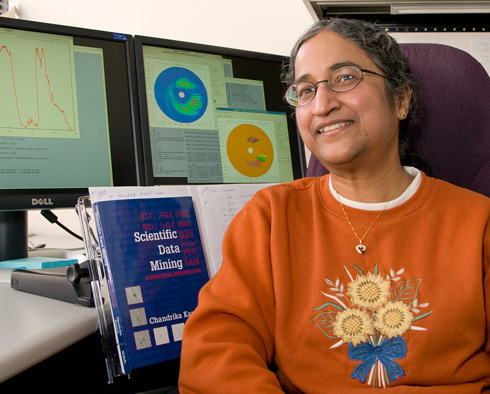
A growing challenge scientists face today is how to extract useful information from the massive amounts of data generated by ever-more powerful computing systems and the increasingly complex applications they run.
In an age of petascale (quadrillions of operations per second) computing, which allows researchers in a broad range of scientific fields to computationally address complex phenomena, data sets are measured in terabytes (trillions of bytes) and petabytes (quadrillions of bytes). Scientists increasingly find themselves confronted by data overload.
"It's no longer practical to manually explore, analyze and understand data," says Chandrika Kamath of the Lab's Center for Applied Scientific Computing (CASC). "It's not just the amount of data but the complexity as well. There are lots of issues."
Drawing on the breadth of her computational and technical experience, Kamath has authored a book offering techniques for addressing the problem of data overload in science and engineering, "Scientific Data Mining: A Practical Perspective," published by the Society for Industrial and Applied Mathematics (SIAM). While the book is unlikely to appear in local bookstores any time soon (though it is available on Amazon), it has proven popular in the research community since its publication in May. "It's selling well, which is quite surprising in this economy," Kamath said. "This is an area a lot of people in science and engineering are interested in."
The target audience for the book includes practitioners of data mining and scientists who need to apply data mining techniques to their research as well as graduate and undergraduate students of the discipline, she says. "The idea of the book was to have a reference where different techniques for data analysis can be found in one place."
In the preface to the book, Kamath defines data mining as "the process concerned with uncovering patterns, associations, anomalies and statistically significant structures in data. It is an iterative and interactive process involving data preprocessing, search for patterns and visualization and validation of the results."
As a field, data mining covers a broad cross section of computational disciplines including image understanding, statistics, machine learning, mathematical optimization, high-performance computing, information retrieval and computer vision, she said. "Data mining techniques hold the promise of assisting scientists and engineers in the analysis of massive, complex data sets, enabling them to make scientific discoveries, gain fundamental insights into the physical processes being studied, and advance their understanding of the world around us."
The multidisciplinary nature of data mining is reflected in Kamath's career experience starting before she came to LLNL when she worked as a software engineer optimizing the techniques underlying search engines for Digital Equipment Corporation. Kamath holds six patents in data mining and has led numerous workshops on data mining for a number of organizations including SIAM. In addition, she is one of three founding editors of the Wiley journal Statistical Analysis and Data Mining , which focuses on practical applications of data analysis techniques.
Kamath served as leader for Sapphire, a LLNL scientific data mining project that earned a R&D 100 award in 2006. The Sapphire project consisted of developing scalable algorithms for the interactive exploration of large, complex, multidimensional data. The tools developed have broad application in plasma physics simulations, remote sensing imagery, video surveillance, climate modeling, astronomy and fluid mix experiments and simulations.
Kamath earned her Ph.D. and master's degree in computer science from the University of Illinois at Urbana-Champaign. She received her B. Tech degree in electrical engineering from the Indian Institute of Technology in Mumbai.
Reflecting on her career, Kamath said she did not set out to pursue data mining but that "opportunities came by." Her specialized knowledge has subsequently provided opportunities for her to apply her skills in a variety of fields and brought her back to interests from earlier in her career — the electricity grid, for example.
"There are areas we previously didn't think of applying data analysis," Kamath said. "We've been looking at wind energy and the challenges related to incorporating increasing percentages of renewable resources on the power grid."
While wind energy may seem simple, the fluctuations of wind make it difficult to manage, she said. "Now we're analyzing weather data to better manage wind resources. This is the kind of analysis we wouldn't have thought of doing in the past."
Data mining will no doubt offer other opportunities to explore emerging fields of research, she said. "It's all fun."