Early COVID-19 mutations associated with later variants
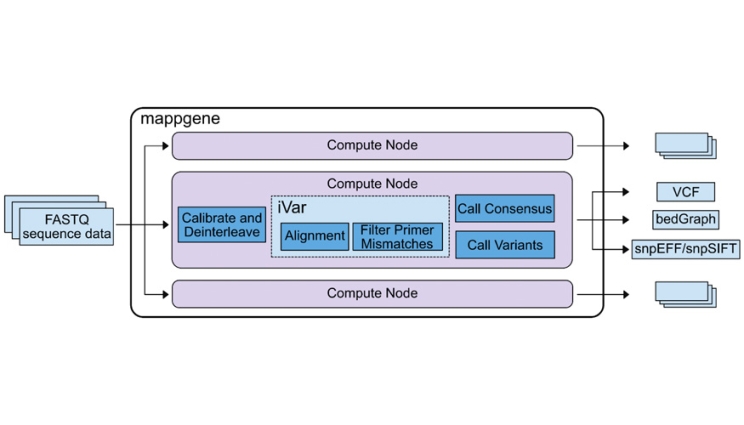
Mappgene pipeline overview. The center box shows the mappgene pipeline workflow, and the other 2 computer node boxes above and below the mappgene node signify that mappgene can run on parallel nodes to process large datasets more rapidly.
The emergence, spread, and evolution of severe acute respiratory syndrome coronavirus-2 (SARS-CoV-2) has been chronicled by the scientific community with greater speed and depth than any other human pathogen due to the advent of widespread genomic sequencing. To facilitate this, numerous websites and dashboards have been created to enable the visualization and contextualization of emerging mutations.
Due to the high mutation rates that characterize the evolutionary dynamics of SARS-CoV-2, deep sequencing is required to capture the genetic diversity of the mutant populations that shape infection outcomes and variant emergence. However, while data on subconsensus (non-dominant/rare) mutations provide a rich source of genetic information about the emergence of viral variants, the genomic sequences used to populate the databases that scientists and public health officials rely on to study the transmission and evolution of SARS-CoV-2 represent the consensus (dominant) sequences detected in a sample.
In a recent study, LLNL and collaborators analyzed 90 COVID-positive, early pandemic clinical samples for the presence and persistence of subconsensus mutations that later emerged in consensus sequences as the pandemic progressed. To do this, the team developed mappgene, a bioinformatics pipeline for high-performance computing designed to process large datasets of deeply sequenced samples and detect low-frequency variants with high confidence. The analyzed results indicated that a subset of mutations, which emerged months later in consensus sequences, were detected as subconsensus members of mutant populations in the early samples.
While the predictive capacity of this information may be constrained by temporal and geographical limitations associated with the samples analyzed, deep sequencing data may be useful for understanding which mutations may emerge in response to environmental changes such as host immune response. Future studies will include analysis of deep sequencing data from samples collected later in the progression of the pandemic to understand if signature mutations for variants of concern/interest can be detected as rare variants prior to detection in consensus sequence data.
[J. Kimbrel, J. Moon, A. Avila-Herrera, J.M. Martí, J. Thissen, N. Mulakken, S.H. Sandholtz, T. Ferrell, C. Daum, S. Hall, B. Segelke, K.T. Arrildt, S. Messenger, D.A. Wadford, C. Jaing, J.E. Allen, M.K. Borucki, Multiple Mutations Associated with Emergent Variants Can Be Detected as Low-Frequency Mutations in Early SARS-CoV-2 Pandemic Clinical Samples, Viruses (2022), doi: 10.3390/v14122775.]
Tags
Bioscience and BioengineeringBiosciences and Biotechnology
Physical and Life Sciences
Featured Articles


